Machine Learning has emerged as a transformative technology with its implications across various industries. It has advanced the way we process and analyze data, enabling systems to learn and improve from experience without explicit programming. While the benefits of Machine Learning are evident, it is crucial to subject it to a critical evaluation to fully grasp its potential advantages and pitfalls. In this blog, we will delve into the key advantages and disadvantages of Machine Learning, highlighting its impact on society, privacy, and decision-making processes.
Understanding Machine Learning:
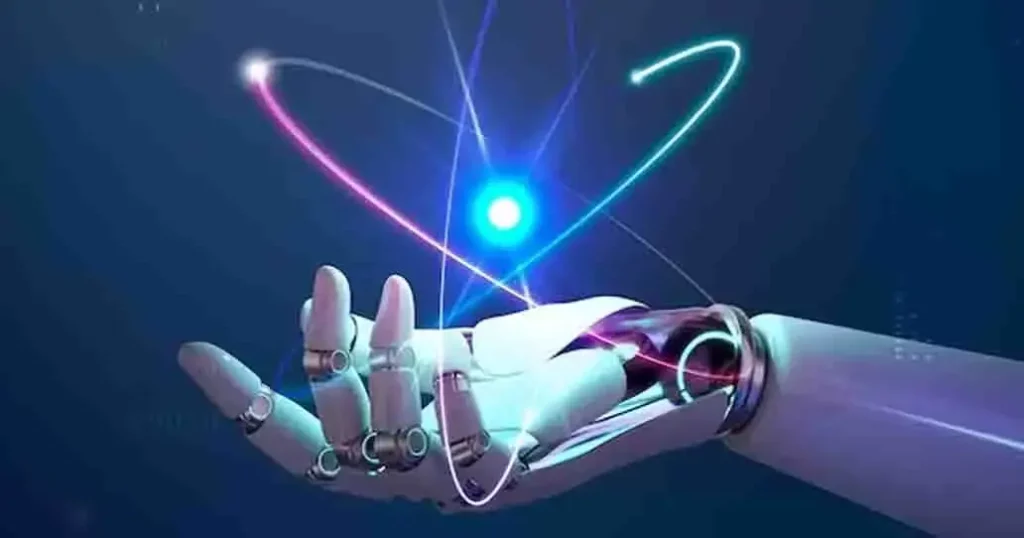
Machine Learning is a subset of artificial intelligence (AI) that enable computer systems to learn from data and make predictions or decisions by developing algorithms and statistical models. It does not require the use of explicit programming language for each specific task. The main goal of Machine Learning is to allow computers to learn and improve their performance on a given task through experience and exposure to data.
In traditional programming, developers write explicit instructions and rules for the computer to follow, providing a specific set of input data and expected output. However, in Machine Learning, the computer learns from examples and data patterns to make decisions and predictions.
Process of Machine Learning:
The typical process of Machine Learning involves the following steps:
Data Collection:
Gathering and acquiring relevant data that will be used to train the Machine Learning model.
Data Preprocessing:
Cleaning and preparing the data to ensure it is in a suitable format for training the model.
Feature Selection:
Identifying and selecting relevant features (attributes) from the data that will be used as input for the model.
Model Selection:
Choosing an appropriate Machine Learning algorithm or model architecture based on the nature of the problem and the data.
Model Training:
Feeding the prepared data into the selected model allows it to learn from the data patterns and relationships.
Model Evaluation:
Assessing the performance of the trained model using various evaluation metrics to determine its accuracy and effectiveness.
Model Tuning:
Fine-tuning the model’s parameters to optimize its performance and achieve better results.
Model Deployment:
Integrating the trained model into real-world applications to make predictions or decisions on new, unseen data.
Advantages and Disadvantages of Machine Learning:
Some advantages and disadvantages of Machine Learning are explained here:
Advantages of Machine Learning:
- Automated Decision-Making
One of the most significant advantages of Machine Learning is its ability to automate decision-making processes. This results in faster and more accurate decisions, reducing human error and bias.
- Pattern Recognition and Prediction
Machine Learning algorithms excel at recognizing patterns and trends in vast datasets. This capability empowers businesses to make data-driven decisions, identify opportunities, and predict future outcomes.
- Personalization
Machine Learning enables personalized user experiences by analyzing user preferences, behavior, and historical data. This personalization enhances customer satisfaction and retention across various industries like e-commerce, entertainment, and marketing.
- Efficient Data Processing
With Machine Learning, vast amounts of data can be processed and analyzed at lightning speed, providing real-time insights and improving operational efficiency.
- Fraud Detection and Security
Machine Learning plays a vital role in fraud detection and cybersecurity by identifying unusual and suspicious activities, thus protecting sensitive information and financial assets.
- Healthcare Advancements
Machine Learning applications in healthcare have shown promise in disease diagnosis, treatment recommendations, drug discovery, and personalized medicine.
Disadvantages of Machine Learning:
- Bias and Fairness Concerns
Machine Learning algorithms are only as unbiased as the data they are trained on. If historical data contains biases, the algorithm can perpetuate these biases, leading to unfair outcomes and discriminatory decisions.
- Overfitting and Generalization Issues
Machine Learning models may overfit the training data, leading to poor generalization on unseen data. This can result in misleading predictions and unreliable insights.
- Data Privacy and Security
The proliferation of Machine Learning also raises concerns about data privacy and security. As models require vast amounts of data to train effectively, protecting sensitive information becomes a challenge.
- Lack of Transparency and Interpretability
Some Machine Learning models, like deep neural networks, are considered “black boxes” as they lack transparency in their decision-making process. This lack of interpretability can be problematic, especially in critical applications like healthcare and finance.
- High Computational and Resource Requirements
Complex Machine Learning models demand significant computational power and resources, making them less accessible for smaller organizations or those with limited budgets.
- Lack of Causality
Machine Learning models can establish correlations but may not provide insight into the causality of relationships between variables, leading to potential misinterpretation.
Conclusion:
Machine Learning undoubtedly offers remarkable advantages across various domains, revolutionizing industries and enhancing our daily lives. However, a critical evaluation is necessary to address its potential pitfalls and challenges. By understanding and mitigating issues such as bias, privacy, and transparency, we can harness the full potential of Machine Learning while ensuring its ethical and responsible use. Striking a balance between the advantages and disadvantages of Machine Learning will pave the way for a future where this transformative technology benefits society at large.